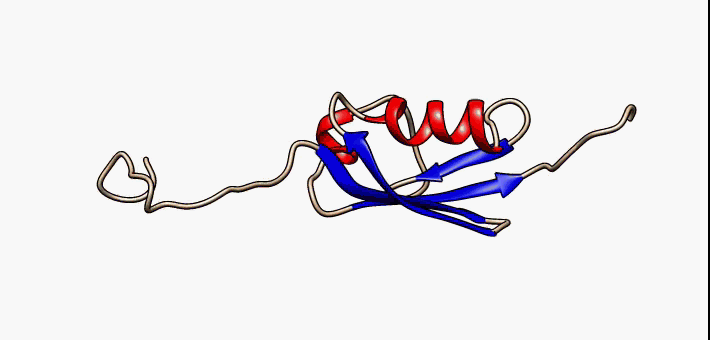
DISPPRED
DISOPRED predicts structural disorder in proteins. It uses an inventive knowledge based approach to train the system, which can the be applied in a predictive manner on novel proteins.
In Detail
DISOPRED2 was trained on a set of around 750 non-redundant sequences with high resolution X-ray structures. Disorder was identified a residues that appear in sequence records but without coordinates. Though an approximation, as coordinates may be missing for other reasons this is a good way to generate a training set where the majority of instances are most likely to represent disorder.
A sequence profile was generated for each protein using a PSI-BLAST search against a filtered sequence database. The input vector for each residue was constructed from the profiles of a symmetric window of fifteen positions.
The data were used to train linear support vector machines (SVMs). The SVM controls overfitting by ensuring that the decision surface separates the two classes with a large margin. An example linear decision surface that separates two classes in 2D (solid line) is shown. The circled points denote the support vectors which lie on the margin (dashed lines).
To avoid unbalanced class frequencies in the training set (a ratio of approximately 19:1) influencing overall accuracy, the training of DISOPRED2 places greater cost on points in the minority (disordered) class than points in the majority (ordered) class.
See the following for more Information
Ward JJ, Sodhi JS, McGuffin LJ, Buxton BF and Jones DT (2004)
Prediction and functional analysis of native disorder in proteins from the three kingdoms of life
Journal of Molecular Biology, 337, 635-645.
About the image on this banner
This helpful image was sourced from the following page of Wikipedia using CC.40. It visually describes conformational flexibility in the protein SUMO-1.